Jawed
Legend
I just love how gigacorps are building their own chips from scratch:
The video is only about 20 minutes and has some nice nuance that you won't get from this article:
Tesla's insane new Dojo D1 AI chip, a full transcript of its unveiling | TweakTown
The article is also missing some slides, which is a shame. 5x GPU in off-chip bandwidth for system-wide networking is a non-trivial factor (timestamped video for the slide I'm referring to):
The video is only about 20 minutes and has some nice nuance that you won't get from this article:
Tesla's insane new Dojo D1 AI chip, a full transcript of its unveiling | TweakTown
The article is also missing some slides, which is a shame. 5x GPU in off-chip bandwidth for system-wide networking is a non-trivial factor (timestamped video for the slide I'm referring to):
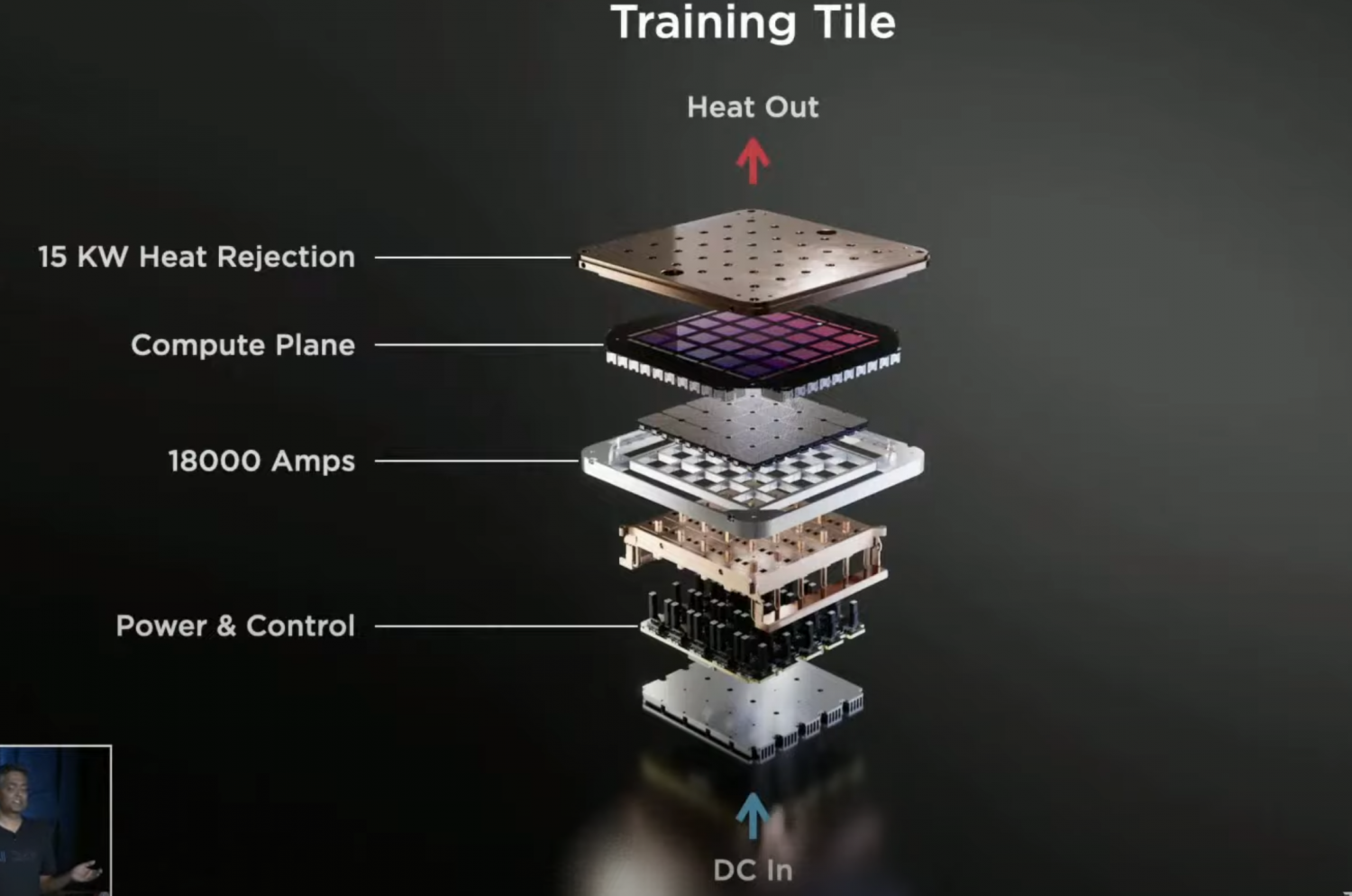
100% of the area out here is going to machine learning training and bandwidth -- there is no dark silicon, there is no legacy support. This is a pure machine-learning machine.
So actual self-driving coming real soon now?it will be the fastest AI training computer -- 4x the performance at the same cost, 1.3x better performance-per-watt -- that is energy-saving, and 5x smaller footprint