because memory is only one (small) part of the power equation. For example, on your beloved MI25 300W accelerator board, HBM2 consumes only 30 watts, ie 10%. The Silicon consumption is still by far the biggest problem and Vega is behind Pascal, whatever says the single AI bench AMD showed us with their established now legendary fake results and using an old version of CUDA. So I will wait for independent results before admitting MI25 is faster than now discontinued P100, which by the way is irrelevant as V100 is now in the market...Why would it need undervolted? That would surely help, but nobody is undervolting in a server market. If anything they simply run cards slower to be more power efficient. The savings with memory types are well established, so feel free to educate yourself before making a fool of yourself like you just did here.
Check your facts. TPU2 is rated at 250W for a single chip. One blade of 4 TPU2 uses redundant 1500W PSUs. If you had ever looked at the MA-SSI-VE heatsink of TPU2 you would have keep quiet. I save you a google search with the picture below:So your reality broke down and you substituted figures to make it accurate? Take 4 chips, disabled three of them, then presumed the one remaining chip still consumed the same amount of power? I suppose that's one way to make 180TFLOPs@250W less than 120TFLOPs@300W.
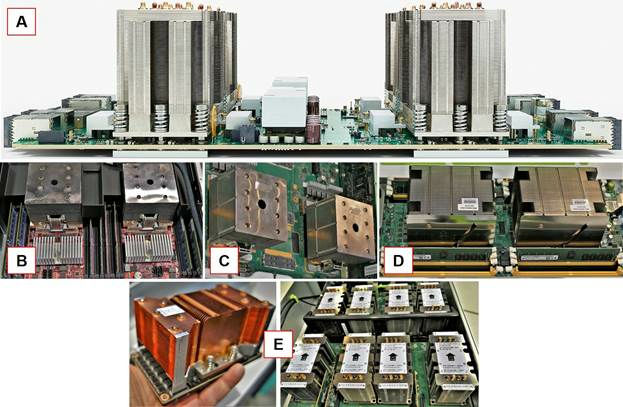
See my reply above ; up to now, we have a single biased compute AI bench presented by AMD on old Nvidia hardware and old software, but you still call it a win ? As for gaming Vega, let's wait for independent AI benches before any definitive conclusion, especially against Volta range, the real competition.I think you're the one in need of a reality check. Compute and graphics are entirely separate areas. Vega is already beating P100 in some tests as expected, yet you feel Nvidia's GDDR5 offerings are superior to even their largest chip? That's just **** stupid, yet you're accusing me of being a fanboy for actually posting accurate information while you link marketing BS? Please show yourself out because this crap is hardly worth the effort of responding.
Then I remind you that the market is not only the single top chip, but customers are buying much more of the smaller cards (from both vendors, ie look at Instinct range). Thus Tesla and Instinct are also available with GDDR5/5x memory, reason of my remark. CQFD
Finally, we agree in one point. I won't neither spend more of my time responding to your nonsens and FUD. I still wish you a nice day :smile:
Last edited: